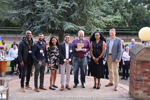
Congratulations to the winners of APM’s Volunteer Achievement Awards 2025
The winners of APM’s Volunteer Achievement Awards have been announced following a ceremony taking place at the Volunteers’ Development Forum in Staffordshire on 26th June.
The winners of APM’s Volunteer Achievement Awards have been announced following a ceremony taking place at the Volunteers’ Development Forum in Staffordshire on 26th June.
Association for Project Management (APM) has once again been attending and exhibiting at Civil Service Live to engage and support project professionals within the civil service.
Tributes have been paid to Professor Stephen Wearne, a highly influential figure in the project profession and Honorary Fellow of Association for Project Management, who has passed away.
The SWWE Regional Network were very pleased to welcome Claire Mills to speak at the event at BAWA on 17 June 2025.
As part of a series of webinars for APM corporate partners and affiliates, Andrew Baldwin, APM’s Head of Policy and Public Affairs, hosted a session focusing on the All Party Parliamentary Group (APPG) for Project Delivery which launched in February.
Projects, programmes and portfolios are essential to the armed forces.
The UK Government has published a new 10-year strategy that sets out its long-term plan for economic, housing and social infrastructure to drive growth.
APM Fellow Gareth Stapleton has been awarded the honour of Officer of the Order of the British Empire (OBE) in the King’s Birthday Honours, for his services to architecture, project and construction management – an honour that reflects his national and international impact.
The project profession is well represented in the King’s Birthday Honours List – the annual list of people being recognised by the British Monarch for their achievements or service to the country.
Association for Project Management (APM) has signed the Armed Forces Covenant, a voluntary pledge which demonstrates an organisation’s commitment to supporting the Armed Forces community, and recognising the value of serving and ex-serving personnel and their families.